ECMWF-ESA workshop on Machine Learning for Earth system observation and prediction
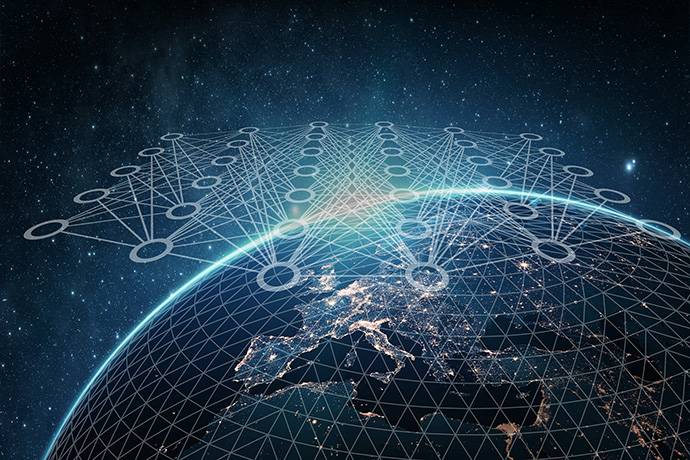
ECMWF | Reading | 5-8 October 2020
Machine Learning/Deep Learning (ML/DL) techniques have made remarkable advances in recent years in a large and ever-growing number of disparate application areas, e.g. natural language processing, computer vision, autonomous vehicles, healthcare, finance and many others. These advances have been driven by the huge increase in available data, the increase in computing power and the emergence of more effective and efficient algorithms.
Earth System Observation and Prediction (ESOP) have arguably been latecomers to the ML/DL party, but interest is rapidly growing, and innovative applications of ML/DL tools are also becoming increasingly common in ESOP.
The interest of ESOP scientists in ML/DL techniques stems from different perspectives. From the observation side, the current and future availability of satellite-based Earth System measurements at high temporal and spatial resolutions and the emergence of entirely new observing systems made possible by ubiquitous internet connectivity (so called “Internet Of Things”) pose new challenges to established processing techniques and ultimately to our ability to make effective use of these new sources of information. ML/DL tools can potentially be useful to overcome some of these problems, for example in the areas of observation quality control, observation bias correction and the development of efficient observation operators and observation-based retrievals.
From a data assimilation perspective, ML/DL approaches are interesting because they can be typically framed as Bayesian inference problems using a similar methodological toolbox as the one used e.g. in variational data assimilation. It can be argued that some of the techniques already common in the data assimilation community (e.g. model error estimation, model parameter estimation) are effectively a type of ML/DL. The question is then, what lessons can the ESOP community learn from the methodologies and practices of the ML/DL community? Can we seamlessly integrate these new ideas into current data assimilation practices?
ML/DL solutions are also being explored for model identification, either in terms of the full forecast model or for specific model parametrizations which are computationally expensive and/or physically uncertain. How to best combine physical knowledge with the statistical knowledge provided by ML/DL approaches is an important and open question. Various types of machine learning technologies have also a rather long history of application in model interpretation and post-processing. The question of how ML/DL can help us extract more value from environmental forecasts is thus a relevant and current one to pose.
An important issue are the uncertainty characteristics of the ML results, and to understand better what physical relations they have been trained on. Many methodologies for both uncertainty quantification and for back-tracing ML output to input features have been proposed, but there is not yet a consensus view. Progress here is needed to improve and better understand reliability of ML results, which is crucial in an operational context.
In the application of ML/DL techniques to ESOP there are still many unanswered questions. The aim of the workshop is to appraise the state of the art of the application of ML/DL techniques to ESOP, to identify the main issues that need to be solved for further progress, and to make a start on charting ways forward. Presenters of the longer talks will be expected to cover not just their own work but also to give a general overview of the subject. Discussions will be facilitated by parallel working groups where the main issues will be discussed in more detail. The output of the workshop will be in the form of working group reports, to be summarised in a technical memorandum or paper.
Registration for the workshop will open in April 2020.
Both oral and poster presentations are encouraged. Attendance that contributes only to the working groups and discussions is also welcome, up to available capacity.
Share