AI4EO Challenge with BIG DIVE
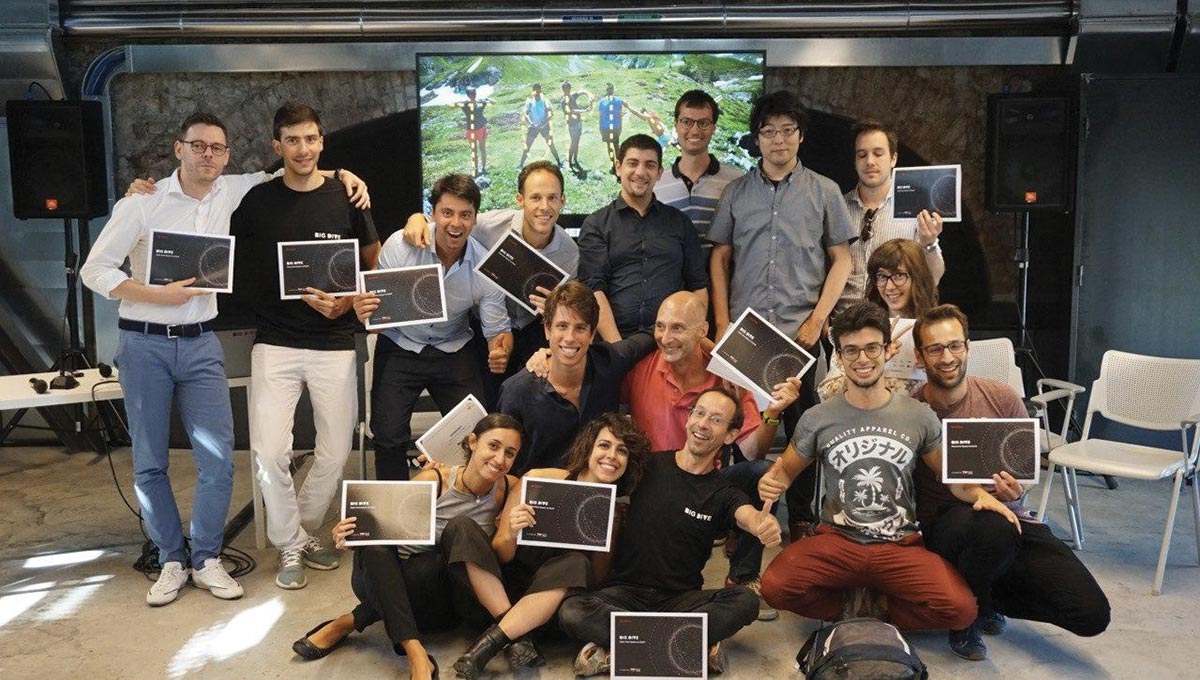
BIG DIVE is an initiative, organised by TOP-IX, ISI Foundation, AXANT offering private and public training on Data Science, Machine and Deep Learning, Data Visualisation, and Data Engineering. The BIG DIVE platform presents itself as an interactive “ street-fighting gym” which puts the raw material – in the form of high value datasets – into the hands of “ambitious smart geeks” tutored and mentored by experts in three key areas: Development, Visualization and Data Science. Courses include lectures by experts in the field and the latest resources and technologies.
In 2019, the focus for BIG DIVE 8 was space data and satellite images.
ESA as one of the main data sponsors collaborated from the beginning to decide the datasets for two of the final projects, ensure the challenges were up to date and relevant and prepare the class to tackle the real-world problems in the space sector and machine learning in general. The three-week training covered the necessary programming and data skills, the best approaches to predictive models, and lectures in the space industry by ESA, Target Detection, Starlab, and forefront research centres. The final week the students, mentored by the teachers, worked and presented four final projects on diverse fields such as urbanization, polar regions, agriculture, atomic clocks and space weather.
One of the projects was the set up of an image recognition challenge with sea ice images a dataset of high-resolution Copernicus Sentinel-1 satellite images on a selected polar area. An ML system for the detection of ice presence and concentration was developed in order to automatically produce an ice map. The group explored the concentration of ice using the ASIP sea ice data set of 26 files in NetCDF format. From that data they extracted matrices and transformed them in order to feed the ML model. The trained a U-net model and evaluate the quality of the learning by using the Jaccard coefficient. Normally, the interpretation of the ice charts is done manually, therefore the project was an important attempt in making the process automatically and faster to allow in the future a scalable approach.
Share