MAPTCHA – a platform where citizen science meets Earth observation for fast emergency responses
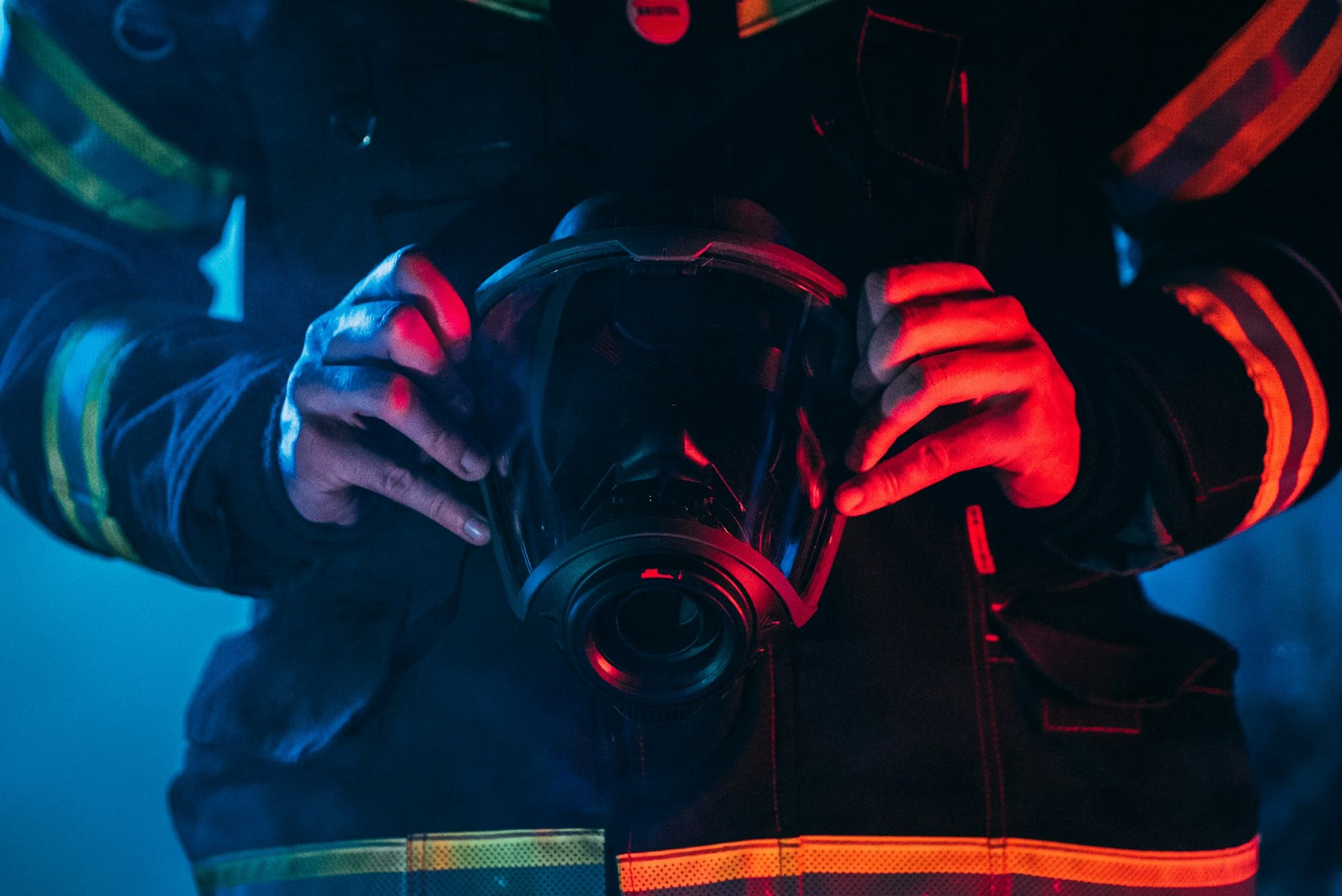
Every second counts when disaster strikes. Whether it is a wildfire or an earthquake, extracting crucial information from satellite data in a fast way is paramount for an effective emergency response. Supported by ESA Φ-lab, the MAPTCHA project will revolutionise the way first responders make informed decisions and potentially save lives during natural catastrophes.
Imagine you are about to sign up for a new service or make a purchase online, and suddenly you face the following challenge – “Select all the images with traffic lights”. Sounds familiar, correct? This is Human CAPTCHA (hCAPTCHA – Completely Automated Public Turing test to tell Computers and Humans Apart), a digital gatekeeper designed to distinguish human users from automated bots.
Now, consider the following scenario: you are looking at a satellite-derived image and you see a fire starting to develop. What if there was a quick way to flag this occurrence, enabling fast responses and informed decisions from emergency teams? This is exactly what MAPTCHA, a citizen science-based project, is trying to accomplish. MAPTCHA – just like “CAPTCHA”, but for “Maps” – is being developed by Osir.io and RSS-Hydro, in collaboration with ESA Φ-lab.
So, let’s take a look at the general MAPTCHA system: it starts by screening natural disaster events from various Internet sources and then collect relevant information such as the area of interest (i.e., the region affected), date of the event and event type (e.g., wildfire) and progression.
Next, the system identifies and retrieves new Sentinel-2 data that are associated with the detected events. These data are pre-processed to convert the multispectral data into false-colour composition that can be better interpretable by the human eyes, tiled in small patches, and then distributed to ‘Human Platform’. This service provided by Intuition Machines allows hCAPTCHA-based annotations, accessible to the entire global population with Internet access. The annotations provided by ‘Human Platform’ are stored and can then be visualised through a mapping tool that enables interactive map exploration.
Annotations are a crucial part of the system. In machine learning (ML) and artificial intelligence (AI), image annotation is the process of labelling images to train AI and ML models. This practice often involves human annotators that assign relevant classes to different entities in an image. For instance, a person can be asked to identify vehicles in a set of images – just like in an hCAPTCHA test. The resulting data from that identification (or annotation) can help train AI/ML models that can recognise and detect vehicles and discriminate them from pedestrians, traffic lights, or potential obstacles on the road to navigate safely.
By taking advantage of the massive volume of crowd-sourced image annotations, MAPTCHA seeks to significantly speed up the process of gathering information on damaged areas, resource allocation and displaced populations, empowering first responders and allowing informed decision making.
Its value goes beyond emergency situations: the massive volume of annotations generated by the platform will serve as a rich training dataset for ML models. This can significantly improve the capabilities of AI-powered Earth Observation applications, leading to advancements in fields such as environmental monitoring, resource management and climate change analysis.
In a collaboration with NASA for the FireCapture project, MAPTCHA is focused, for now, on early fire detection. Several Copernicus Emergency Management Service wildfire events in Bolivia, Spain, Chile and Mexico were used as study cases.
For the events in Chile, for instance, preliminary tests showed that around 60 annotations per minute were being collected, up to a total of 73 000 annotations. These tests showed that automated bots have difficulties accessing and interpreting Sentinel-2 imaging after the pre-processing step, as compared with what happens when a bot tries to identify traffic lights, demonstrating the reliability and added value of MAPTCHA.
“MAPTCHA can do both: adapt rapidly and process very large amounts of Earth Observation data to provide actionable insights for first responders,” says Ron Hagensieker, Founder of Osir.io. “Essentially, this project represents a significant leap forward in Earth Observation, offering a powerful tool not only for crisis response, but also to proactively safeguard our planet for the future generations.”
Guy Schumann, Founder and CEO of RSS-Hydro also states that “Rapid and accurate data extraction from satellite imagery is crucial during catastrophes such as wildfires, floods or earthquakes. By harnessing the collective intelligence of a vast network of users, MAPTCHA demonstrated how online antibot technology can boost Earth Observation data uptake, with huge potential to optimise disaster response.”
“The MAPTCHA initiative paves the way for a future where citizen science and AI converge to unlock the full potential of Earth Observation data”, comments Nicolas Longépé, Earth Observation Data Scientist at Φ-lab. “By facilitating the access to image annotation and continuously improving AI models, we gain a deeper understanding of our planet, developing sustainable solutions for the challenges we currently face”.
To know more: ESA Φ-lab, Osir.io, RSS-Hydro
Photo courtesy of Unsplash/Nejc Soklic
Share