Get ready for the ESA-ECMWF workshop on Machine Learning for Earth System Observation and Prediction
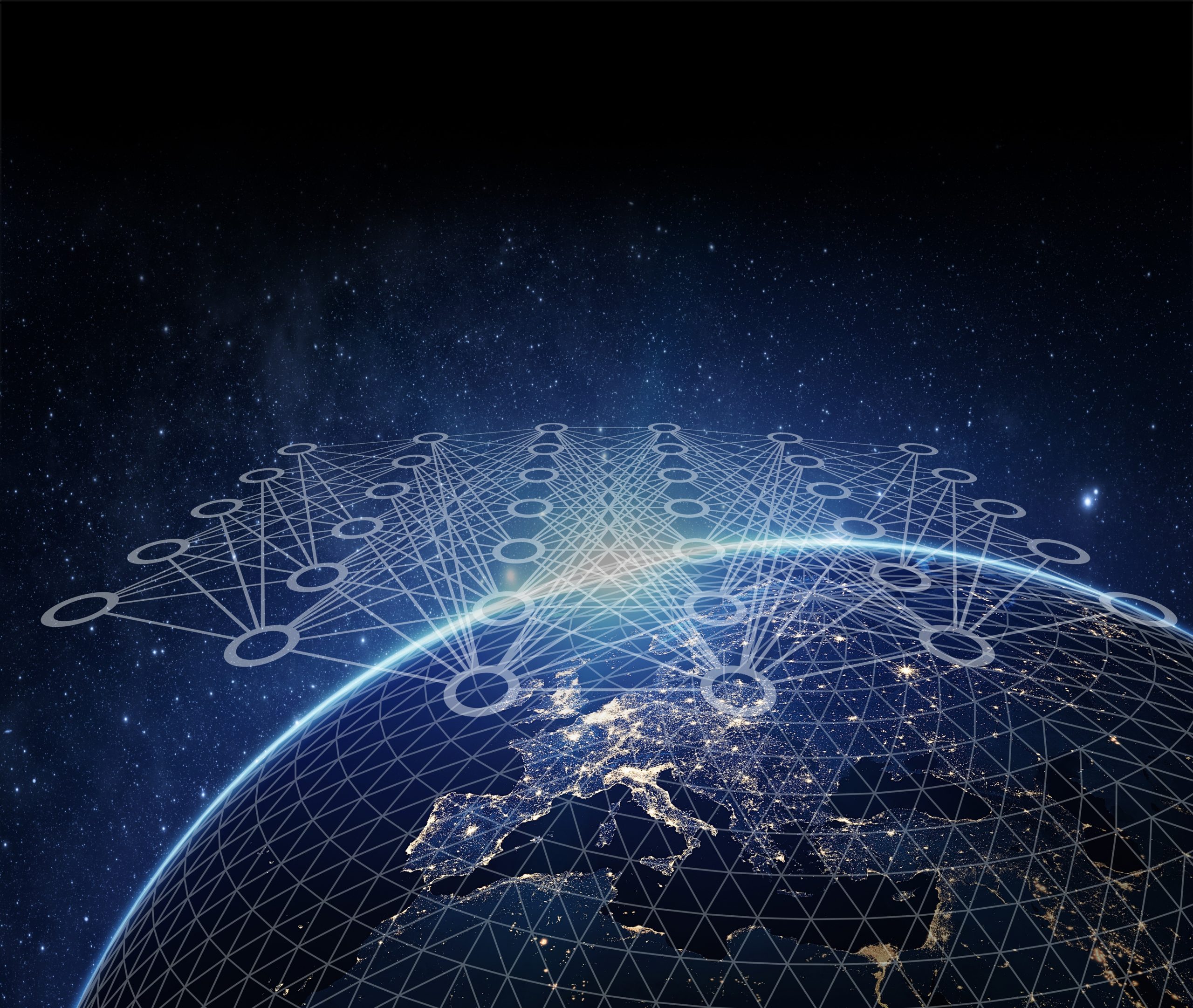
ESA and ECMWF are organising the second joint workshop on Machine Learning for Earth System Observation and Prediction that will be hosted from 15 to 18 November in an hybrid format. The call for abstracts is currently opened until 28 July. Participants registrations will start in August 2021. Submit your proposals.
The advantages of Machine Learning/Deep Learning (ML/DL) Artificial Intelligence techniques have been proven in a wide range of applications such as image recognition, traffic prediction, self-driving vehicles, and medical diagnosis. These techniques have also gained popularity within the Earth System Observation and Prediction (ESOP) community due to their ability to improve our understanding and prediction capabilities on the Earth’s complex and wide-scale dynamics.
Supported by the continuous increase in computing power, these techniques are valuable to automatically process and analyse a large range of available data but they still present some limitations, as for example the case of DL methods that need large amounts of curated and labelled data.
The workshop on “Machine Learning for Earth System Observation and Prediction” from 15 to 18 November, organised by ESA and ECMWF, will aim to demonstrate where and how this fusion between traditional ESOP techniques and new ML/DL methods reached a remarkable impact. It will also help to identify the remaining issues to be further explored. Presenters will demonstrate their contributions to this challenge and expand the discussion to provide a general overview of the subject.
After the first three days, covering the state-of-the-art, the working groups will discuss in parallel the current limitations and suggest how to advance so as to extract more value from this powerful fusion.
The output of the workshop is in the form of working group reports, which will be then summarised in a technical memorandum or a paper.
Abstracts can be submitted online until 28 July 2021. Participants registrations will start in August.
To know more: dedicated event website, ESA Φ-lab, ECMWF
Share