World breakthrough in onboard AI model training presented by Φ-lab at IGARSS
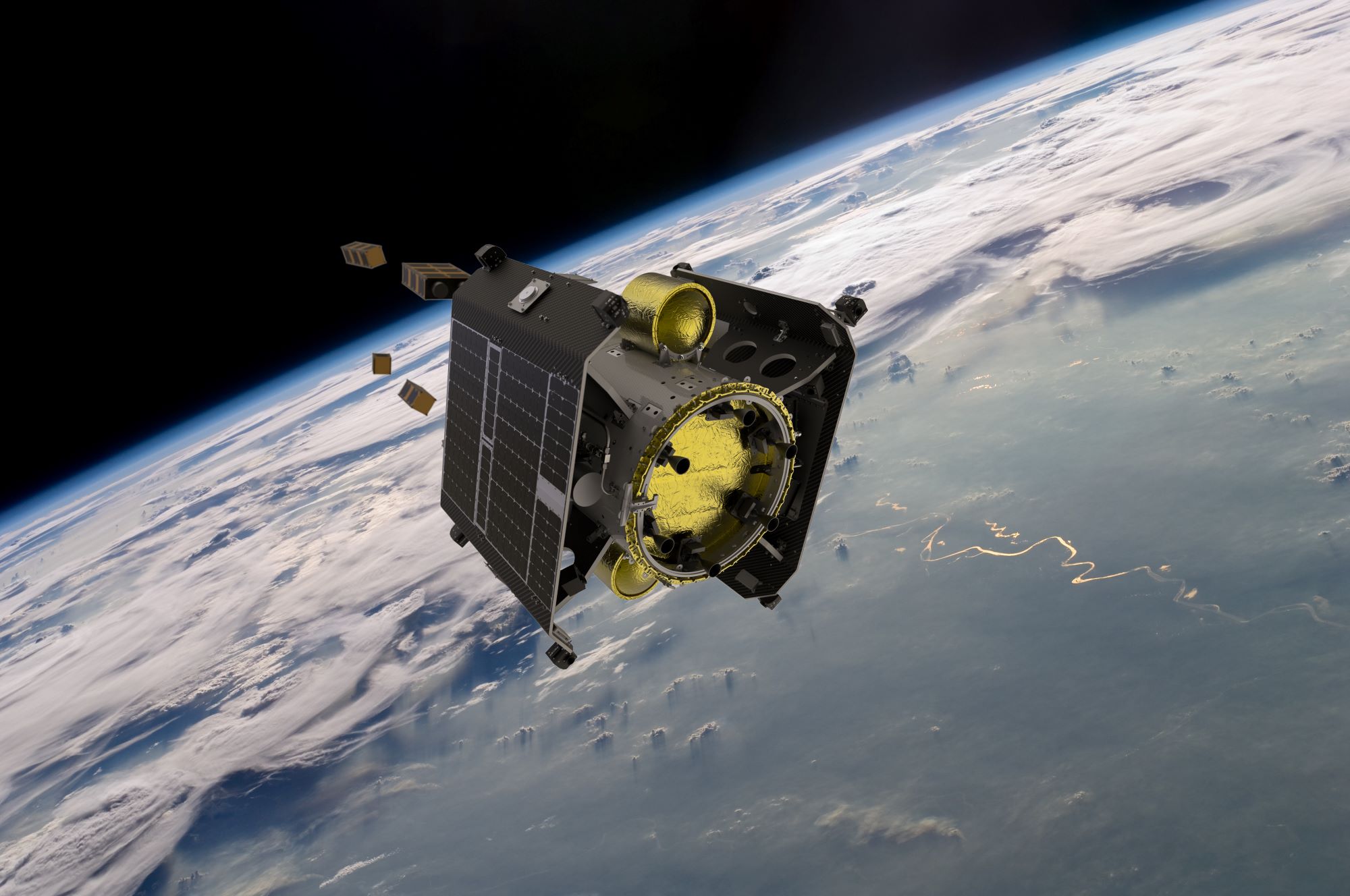
At the International Geoscience and Remote Sensing Symposium (IGARSS) on 21 July, ESA Φ-lab presented the results of groundbreaking research in artificial intelligence (AI) aboard Earth observation satellites. Carried out by Oxford University and Trillium Technologies in collaboration with Φ-lab, the research successfully trained a cloud-detection Machine Learning model while in flight on a D-Orbit ION mission.
ESA has given considerable attention in recent years to Cognitive Cloud Computing in Space (3CS), with such initiatives as the Φsat missions and the 3CS Call for Ideas helping to push the boundaries of enabling ever-increasing computational power onboard Earth observation (EO) satellites. The D-Orbit Dashing Through the Stars mission was launched in January 2022 and included a number of service tests funded by ESA. Among these was a customisable Machine Learning (ML) payload which allows models to be uploaded, updated and run directly on the satellite.
Despite the models running on the satellite, model training would normally take place on the ground due to the computational load and the enormous datasets required. In a world first however, Trillium Technologies and Oxford University’s Department of Computer Science have trained one such model directly onboard during the D-Orbit mission. This development has momentous implications for remote sensing, not only in Earth Observation but also in deep space exploration, as onboard training allows models to adapt autonomously to both changing conditions in space and sensor calibration drift. This is all the more important when the communication channel between the spacecraft and ground is limited due to distance and/or bandwidth.
To overcome the resource constraints of onboard training for ML models, the research group adopted an innovative approach involving an initial ML model known as RaVAEn. This model specialises in efficiently compressing EO images to just a few kilobytes, reducing the data to a ‘latent space’ representation. The key advantage of this methodology lies in the ease with which the constrained satellite hardware can handle the compact latent space. Unlike the original large and data-intensive imagery, the compressed representation is significantly smaller and more manageable.
The full paper on the research will be published soon at ieeeigarss.org.
“RaVAEn first compresses the large image files into a vector of 128 numbers, keeping only the most relevant and informative content,” explains Vít Růžička, the project lead for Oxford University and a former Φ-lab visiting researcher. “By employing RaVAEn to compress the images, a second tiny ML model can work with the compressed latent space and perform onboard training and cloud detection without overwhelming the satellite’s resources.”
Following this initial demonstration of onboard training capabilities with base-level cloud detection, model development will now continue towards more challenging feature detection.
ESA data scientist Nicolas Longépé is leading the research on edge computing at Φ-lab and gave a talk on the results at IGARSS: “This activity received an enthusiastic response at the symposium, with the success of the RaVAEn compression capabilities opening avenues for rapid, computationally efficient training of models directly on the satellite. The results are also significant in terms of the federation aspect of 3CS, as we envisage the enticing prospect of intelligent, autonomous constellations with compressed but reliable data being exchanged within the fleet.”
To know more: ESA Φ-lab, ESA InCubed, IGARSS, Trillium Technologies, Oxford University Computer Science
Main image courtesy of D-Orbit. Copernicus Sentinel-2 imagery courtesy of ESA and processed by Vít Růžička
Share